Thoughts on the future of software.
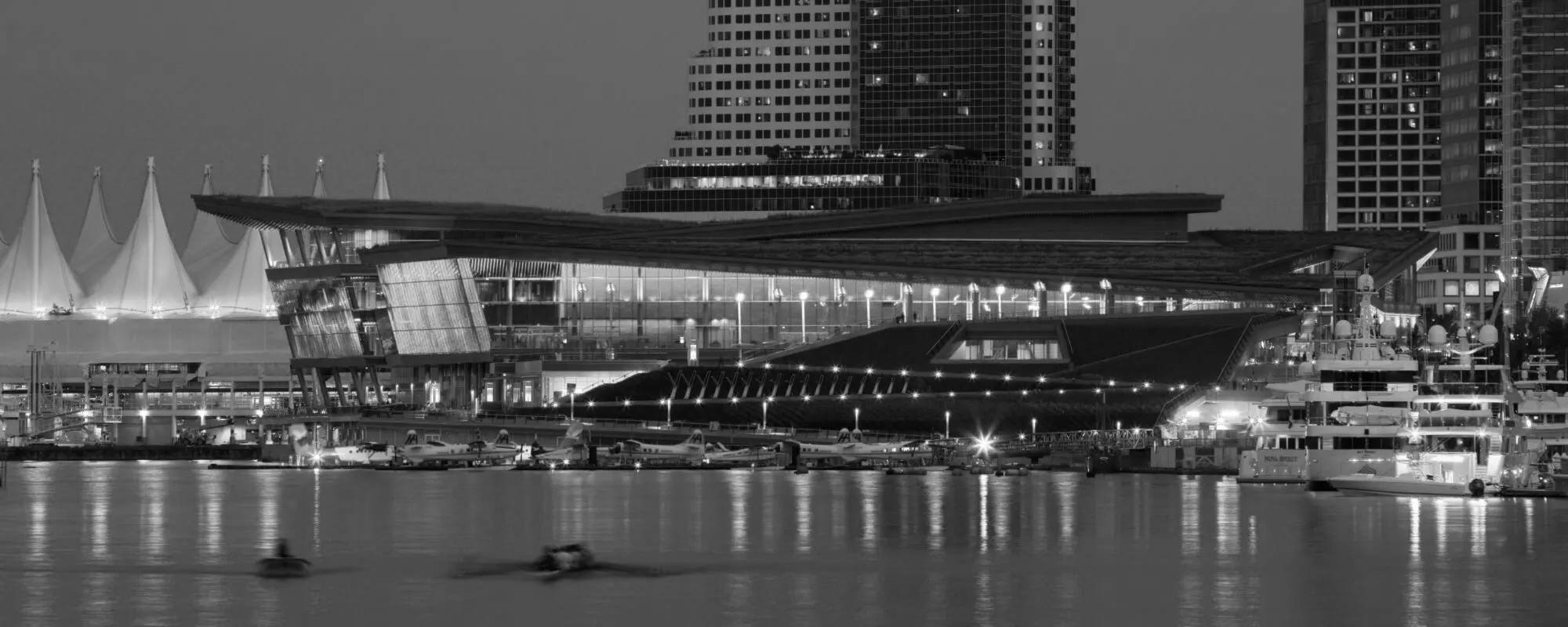
NeurIPS 2024: Beyond Scale
Jack Hogan2024-12-21
Last week, we joined thousands of researchers, engineers, entrepreneurs and investors in Vancouver for NeurIPS 2024—the biggest iteration of the conference yet. The headlines may have been dominated by Ilya Sutskever's somewhat sensationalist The mood at the conference was one of genuine optimism. What could have been an existential moment for academic AI research—with compute requirements spiralling beyond reach—has instead become a catalyst for innovation. Presentation after presentation demonstrated how carefully designed systems, despite using orders of magnitude less compute, could match or exceed the performance of frontier models in specific domains. The successes came from every angle: novel post-training techniques, sophisticated inference strategies, adaptive optimisation methods. The era of innovation in AI, it seems, is just beginning.In this post, we'll share our key takeaways from the conference, focusing on three themes that we believe will be crucial in the coming year: Post-training: Beyond Basic Instruction TuningWith pre-training delivering diminishing marginal returns, much of the conference's focus centred on refining and optimising the post-training pipeline. The contrast with just a year ago was striking, with the basic instruction-tuning techniques that made ChatGPT possible (i.e., A clear recipe has emerged and is thoroughly described in, for example, the The basic outline of the post-training recipe may be settled, but we saw a remarkable diversity in how different teams are implementing and specialising each component. Several papers particularly caught our attention:Direct Q-Function OptimizationProcess Reward ModelSelf-play Preference OptimizationAsynchronous RLHFEach of these innovations represents a different way to extract more performance from existing architectures—better modelling of the reasoning process, denser feedback signals, more nuanced preference structures. For teams like ours working on specialised applications, this is particularly encouraging—with the right post-training pipeline, even relatively small models can achieve remarkable performance in specific domains.Test-time Compute: From Scaling Training to Scaling InferenceJust as post-training techniques have evolved beyond basic instruction tuning, we're seeing a similar evolution in how the community thinks about inference. What started with simple prompt engineering and few-shot learning has developed into a sophisticated toolkit of techniques for extracting better performance at inference time: ranking and merging multiple generations, chain-of-thought prompting, self-refinement loops, and even test-time fine-tuning of the model weights. Several researchers demonstrated how relatively small models with clever inference strategies could match or exceed the performance of much larger models using basic greedy decoding.This shift toward inference-time optimisation raises interesting questions about how we evaluate AI systems—it no longer makes sense to evaluate performance based on a single forward pass completion. Several talks highlighted the need for new benchmarking approaches that consider the full cost-benefit tradeoff, including inference-time compute costs or FLOPs alongside raw performance metrics.The range of inference-time techniques being explored is remarkable:Input augmentation through more sophisticated prompting and in-context learningOutput augmentation via chain-of-thought reasoning and multiple samplingHybrid approaches that combine multiple models or incorporate external toolsTest-time model adaptation, including fine-tuning based on specific inputsOne paperOther papers that caught our attention were:Divide-and-Conquer Meets ConsensusDecompose, Analyze and RethinkEfficiently Learning at Test TimeSystem 2 Reasoning: Beyond Pattern MatchingSunday featured an entire workshop dedicated to System 2 reasoning, reflecting growing interest in moving beyond the pattern-matching capabilities of current AI systems. Throughout the day, researchers explored the intersection of LLM reasoning and cognitive science, examining how machine "thinking" fundamentally differs from human cognition.ISeveral talks explored how we might bridge this gap. A particularly interesting thread focused on the relationship between neural networks and symbolic reasoning. While traditional "hybrid" approaches that combine neural and symbolic systems have shown promise, they often inherit the scalability challenges of symbolic AI. More recent "unified" approaches that embed symbolic structures directly within neural architectures are promising, potentially offering the best of both worlds.For example, The discussions repeatedly returned to a central theme: while language is a powerful tool for communicating reasoning, it might not be the optimal medium for A real highlight of NeurIPS was seeing Agemo explicitly mentioned in François Chollet's keynote talk as one of the startups actively contributing to this space!Looking AheadThe themes that emerged at NeurIPS 2024—sophisticated post-training pipelines, intelligent use of inference-time compute, and a renewed focus on reasoning capabilities—suggest an exciting direction for AI research. Rather than pursuing raw scale, the field is developing more nuanced approaches to extracting intelligence from our models. The resulting systems may be more complex, combining multiple techniques and requiring careful orchestration, but they're also more capable, reliable and efficient.For us at Agemo, these developments are particularly encouraging. Our thesis has always been that truly intelligent software creation requires more than pattern matching—it demands the ability to reason systematically about requirements, architecture, and correctness.We're excited to be part of this evolving conversation about the future of AI and software development. As always, we’d love to hear your comments and feedback. Follow us on
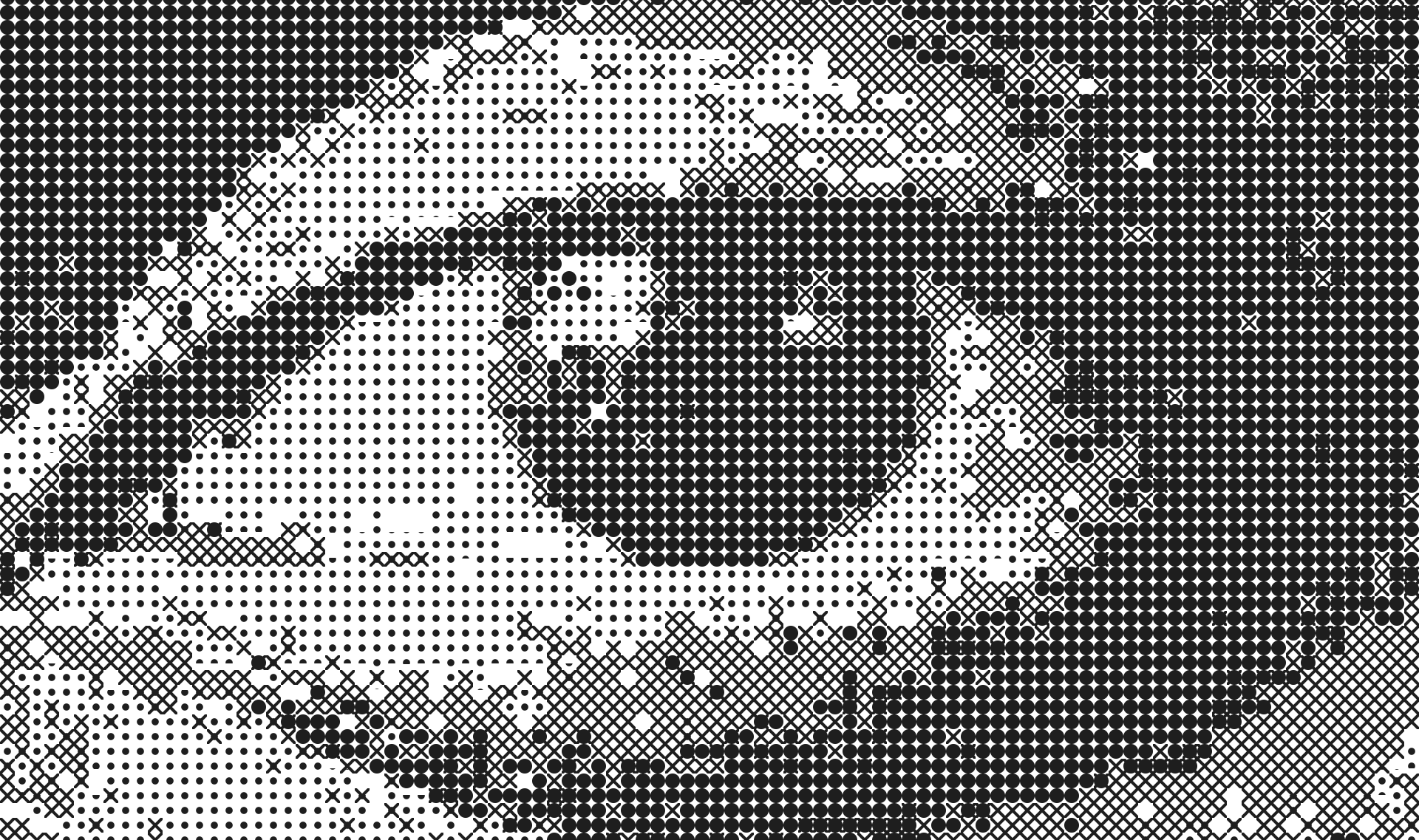
Summer of ARC-AGI: Part 2
Jack Hogan2024-12-04
Part 2: Open Sourcing an Autonomous ARC SolverIn From Research to Open SourceOur internal solver, which managed to solve 85% of the ARC public training set, was built on repurposed proprietary infrastructure from our core product, CodeWords, that would not be practical to release. Instead, we've developed a new implementation that captures the core ideas while being more accessible and extensible. Built using We believe this will be a valuable resource for the ARC research community—the interactive notebook format makes it particularly easy to explore different solving strategies or experiment with new primitive operations, while the modular design allows researchers to focus on specific components without needing to modify the entire system.Architecture OverviewThe solver follows a three-stage pipeline that mirrors how a human might approach an ARC task:Task DescriptionSolution GenerationIterative RefinementEach stage of this pipeline is designed to be modular and extensible. For example, in the description stage, we implemented two different strategies: the solver can use either a "direct" approach (analysing all examples at once) or an "indirect" approach (analysing examples independently, then synthesising the patterns). Many more different strategies or modifications could be considered and easily incorporated into the solver.Key Design FeaturesAt the heart of the solver lies our object-centric framework, allowing solutions to be expressed in terms of high-level objects (`Rectangle`, `Line`, `Bitmap`, etc.) and transformations rather than raw grid manipulations. This abstraction layer makes solutions more interpretable and closer to human reasoning patterns.Caution is always necessary when designing a system that can execute LLM-generated code. Our solver includes highly prescriptive prompts defining the context within which Claude’s proposed solutions should operate. Before any code response is executed, it is parsed and validated to contain classes with the desired method names and types. Only then is it run in an isolated python subprocess with a restricted set of imports and file access.Performance hasn't been overlooked either. The solver leverages `asyncio` for concurrent API calls and multiprocessing for parallel solution testing, making it efficient even when exploring multiple solution paths simultaneously. This becomes particularly important when scaling up to larger sets of tasks or when experimenting with different solving strategies.Research ApplicationsWhile our open-source solver uses Claude instead of fine-tuned models, its modular design makes it valuable for ARC research in several ways:Prompt EngineeringTraining Data GenerationFramework/DSL DesignLooking ForwardBy open-sourcing this solver, we hope to encourage more research into neurosymbolic approaches to abstract reasoning. The ARC challenge remains far from solved, and we believe that exploring the intersection of language models, symbolic reasoning, and object-centric representations will be crucial for progress toward AGI.Stay tuned for Part 3, where we'll dive deeper into how we trained models using solver-generated training data, including preference fine-tuning and inference optimisations. In the meantime, play around with our solver and let us know what you think on
Introducing CodeWords Beta, the idea-to-software platform
Aymeric Zhuo2024-11-21
In the last two years, we’ve seen code autocomplete products enable developers to write code faster while non-developers are still left with no-code platforms with known limitations: steep learning curve, limited functionalities, and lack of flexibility. It can take anywhere AI was supposed to lower the barrier to entry to creating software. It has actually widened that gap between developers and non-developers. At Agemo, we want to make the impossible possible: enabling non-developers to create software. From their own words. From an idea to a ready-to-use tool. In minutes.Introducing CodeWords, the idea-to-software platformToday, we are excited to open up the Beta of CodeWords, an AI-native platform to turn your software ideas into working software. “It's like a MidJourney for software”.CodeWords is powered by our proprietary reasoning engine, built to reason like a team of expert software engineers and implement based on your requirements.On CodeWords, you can build Let’s run through the experience.Draft your SpecWhen building on CodeWords, imagine you’re speaking to your development team in your own words to build a “function spec”. Imagine the spec as the document capturing your project requirements. More literally, this is like a project brief.Build in minutes, not weeksYou can provide as little or as much detailed as you’d like and the function spec will be updated for you at each time. Ready to build?The building time is a key metric we monitor and we ensure CodeWords can build functions under ten minutes.Publish it, in one-clickHappy with the build? A user interface is automatically generated for you but you can also consume it as an API.Can I build anything today?In its current iteration, CodeWords is particularly good at document processing and data extraction use cases, and we are planning to expand to new use case verticals soon.We’ve been thrilled to see our early users building tools such as an earnings report tabular data extractor into CSV tool, a research paper into PowerPoint slides converter tool or a voice transcription and Q&A tool. Try them for yourself:Slide Deck Analysis for Question AnsweringPDF Tables to Excel ExtractorYoutube URL to Blog ConverterThe future aheadWhat we are excited by is the creativity and the level of personalization people have for the software they want. It is a validation of the future we envision: where we are not bound by skill or expertise, but only by our imagination. Where the human remains in the loop but focuses on the what, not the how. That means crafting the perfect requirements. In ten years, code will become a forgotten artefact in ten years – because we will be able to synthesize any software we need.As we open CodeWords to everyone, we would love to hear from non-developers such as project managers, analysts, and designers on what sort of tools they would like to build but have never been able to.Sign upTalk to us about your software needsFor businesses, talk to us about the workflows you are currently outsourcing and the menial tasks slowing down your operations.Companies often have to conform their processes to the constraints of third-party tools, which can stifle efficiency and innovation. At Agemo, we believe every company has a distinct DNA and way of working, and tools should adapt to the unique needs of each team – not the other way aroundWith Codewords, teams are able to build their own internal tools that align seamlessly with their existing processes.Fill out

Summer of ARC-AGI Part 1
Jack Hogan2024-11-08
Part 1: Framing the Problem and Shaping the SolutionAt Agemo, we’re on a mission to revolutionise software development through AI. We believe in empowering people to create sophisticated software solutions without needing deep technical expertise. CodeWords, our core product, is a platform that transforms ideas into deployed software, marking our first step toward that ambitious mission. From natural language user prompts, CodeWords plans, builds, tests and deploys fully functional backend functions.In this blog post, we’ll discuss how our research efforts that make this possible—including neurosymbolic reasoning, reinforcement learning and process-guided search—led us to take on an unexpected summer side quest: tackling the ARC-AGI challenge.The ARC ChallengeThe ARC (Abstraction and Reasoning Corpus) challenge was first introduced by François Chollet, a researcher at Google and the creator of Keras, in his 2019 paper, “On the Measure of Intelligence”. Chollet specifically designed the dataset to test an AI system’s true While modern AI systems excel at most benchmarks and may superficially appear to perform in-context learning and generalisation, ARC exposes their reliance on memorisation and interpolation. Even state-of-the-art AI systems such as OpenAI’s o1 model Earlier this year, ARC caught our attention when Mike Knoop (co-founder, Zapier) teamed up with Chollet to launch a public competition for the ARC benchmark. The dataset consists of a collection of unique Most ARC tasks, such as the example shown, are extremely easy for humans to solve. We do so without requiring any knowledge or experience beyond basic visual understanding and what Chollet refers to as Why We Joined the ChallengeAt Agemo, our research team is constantly working to improve the core intelligence powering CodeWords. Although software may seem pretty far removed from ARC grid puzzles, our focus areas—reasoning, knowledge distillation, reinforcement learning from machine feedback (RLMF), and inference-time optimisations—align closely with the skills required to tackle the ARC-AGI benchmark.When we recognised that many of the building blocks for a neurosymbolic ARC solver were already in place within our existing system, the overlap between ARC's challenges (inductive reasoning, planning, skill acquisition, compute efficiency) and our research agenda was too compelling to ignore.Framing the Problem: Beyond Deep Learning and Discrete SearchThe first step in tackling ARC is deciding on a broad solution strategy. As François Chollet himself "We have two approaches that have basically no overlap, that are doing quite well. They're very much at two opposite ends of one spectrum. On one end, you have these extremely large banks of millions of vector programs, but very shallow recombination, simplistic recombination. On the other end, you have very simplistic DSLs, 100-200 primitives, but very deep, very sophisticated program search. The solution is going to be somewhere in between. The people who are going to be winning the ARC competition and making the most progress towards near-term AGI are going to be those that manage to merge the deep learning paradigm and the discrete program search paradigm into one elegant way."This sentiment aligns perfectly with our research thesis at Agemo. We believe that automated software generation won't be solved by purely symbolic program search, nor by end-to-end training of hyperscale deep learning models. The answer lies in a neurosymbolic approach, where a deep learning model (in our case, an LLM) guides a symbolic reasoning engine.Shaping the Solution: A Flexible, Object-Centric ApproachWith our broad strategy in place, we needed to define or constrain the space of programs in which our system will search for candidate solutions. Many approaches to ARC involve creating a Domain-Specific Language (DSL) with primitives tailored to solve ARC tasks. Michael Hodel, of the currently leading team, MindsAI, created and The function takes an input array and, through repeated function composition from the set of 160 primitives, arrives at a transformation to the corresponding output grid.While it was tempting to piggy-back on this hard work and adopt an existing DSL like Hodel's, we wanted our solution to Our preferred approach—just like our approach to software reasoning—is to provide a framework or environment within which we we can train an LLM to reason about the target domain. ARC-specific DSLs like the above, in an effort to explicitly encode extremely domain-specific logic, often become overly restrictive and abstruse. While this isn't a problem for discrete program search systems, our intention is to guide an LLM to generate candidate solutions expressed in a DSL. We're strong believers that to maximise an LLM's chance of success, we should let it use the tools with which it is most familiar — why force an LLM to use a completely novel grammar and syntax when it has already been pre-trained to excel at manipulating 2d arrays using numpy?Inspired by the "object-centric" approach to modelling ARC tasks of Our framework revolves around “grid models” for a given ARC task:An input grid model parsimoniously describes the space of valid input grids for that task. It declares the variable and invariable properties of the grids in that task; e.g. the presence of certain shapes or patterns, their colours, sizes, etc.An output grid model does the same for output grids, with certain properties declared in relation to the input grid model.We implemented this using Pydantic models, which provide an elegant way to parse and validate grid arrays.To demonstrate, let’s consider again the example grids for task 4c5c2cf0. Based on this image, an input grid model for this task should encode the information that valid input grids are all 14x14 in size, have a black background and contain two coloured shapes. In the below example input model, we declare exactly that. The powerful feature is the ability to easily define a class method that will automatically parse a numpy array into an instance of this grid class.An output grid model can then be used to declare how the corner shape is copied and reflected around the central shape to form a symmetrical pattern.The transformation logic is encoded in the from_input class method, which can leverage simple numpy manipulation methods (e.g. np.flip) to transform an arbitrary input instance into a predicted output grid.The framework was carefully designed with our neurosymbolic approach in mind. It strikes a balance—imposing enough constraints to allow us to symbolically verify proposed solutions (testing both input and output grid reconstruction), while not restricting expressivity and limiting the search space of solutions by enforcing a strict DSL.Our initial set of just 10 core primitives (shapes like Rectangle, Line, and Bitmap, with a couple of useful helper functions, e.g. ShapeExtractor and PatternMatcher) proved surprisingly effective. When we ran v0 of our neurosymbolic solver (using GPT4o) against a random subset of 100 tasks from the public evaluation set, it generated correct solutions for 18% of tasks. When we ran a version of the same solver that uses Hodel’s DSL, it correctly solved 16%.Looking Ahead: Skill Acquisition and Guided Program SearchWhile the ARC competition is ongoing, and we'll share more explicit details in future posts, we want to highlight a key feature of our approach: the ability to expand the set of primitives on the fly. Our automated solver encourages the LLM to define new primitives when it can't solve a task with the existing set. Through an iterative process of running our solver and fine-tuning on correct solutions, we were able to generate valid solutions to >85% of ARC training tasks completely autonomously, using a seed set of only 3 hand-written example solutions.In Part 2 of this series, we’ll dive deeper into the automated solver that we’ve built and explore how the various components work together to tackle unseen tasks. After that, we’ll discuss how we’ve implemented RLMF (Reinforcement Learning from Machine Feedback) in our system, (including hacking vLLM and implementing DPO (Direct Preference Optimization) for vision models), learning from incorrect predictions, and our investigations into inference-time performance improvements, navigating the exploration/exploitation tradeoff using Q-learning.By pushing ourselves to tackle this fun yet deceptively difficult challenge, we’re not just competing in a benchmark—we’re advancing the core technologies that power our mission to democratise software development through AI.Stay tuned as we continue to share our journey and insights from our Summer of ARC-AGI!
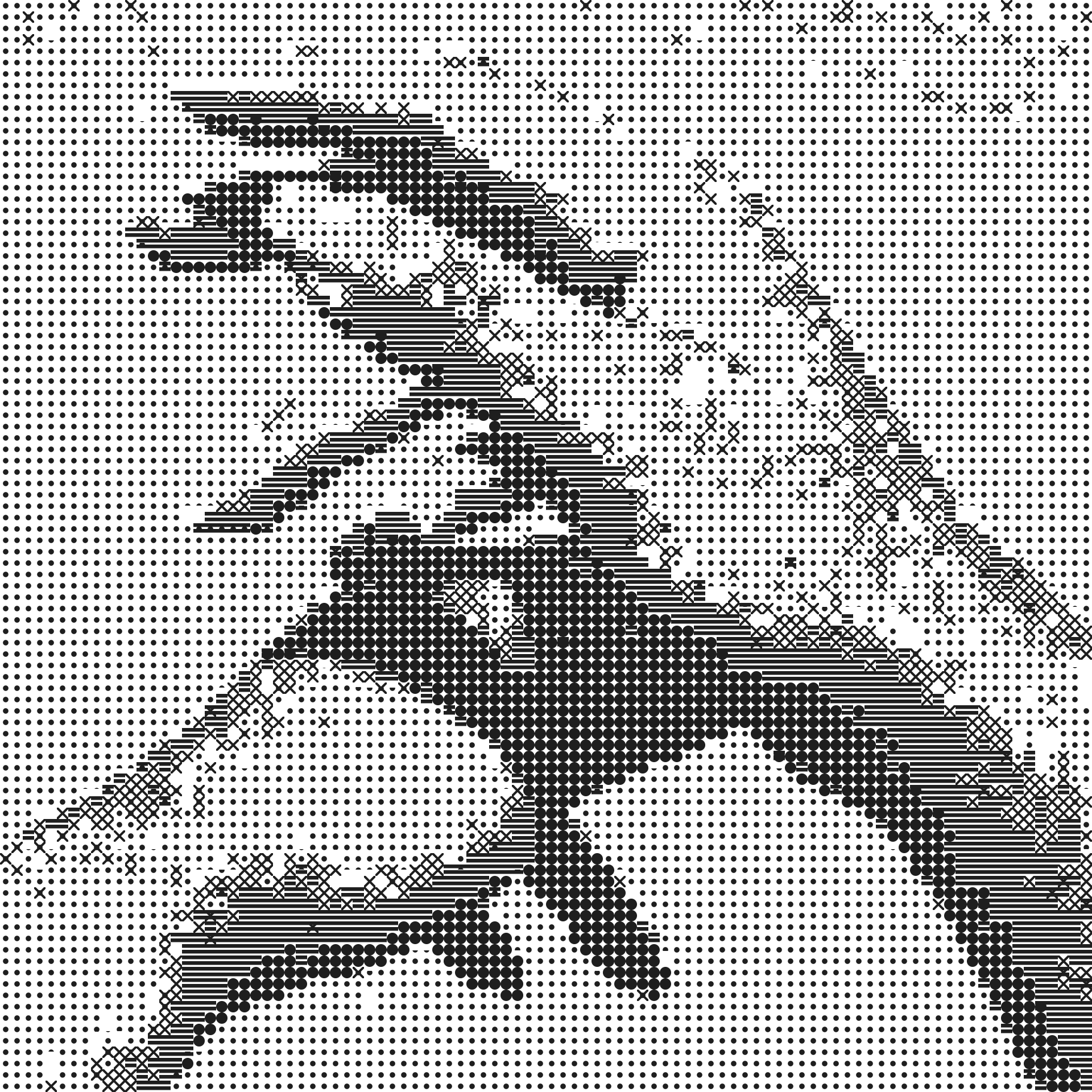
When Purpose Finds Its Pioneers
Emelie Holgersson2024-11-07
Great ideas often come from unexpected places. For Agemo founders Osman Ramadan and Aymeric Zhuo, the journey to building AI that could change the fundamentals of software development began in a Sudanese village and a family grocery store in French Guiana.Their paths – through Cambridge, Microsoft, École Polytechnique, and TikTok – converged on a simple but powerful mission: making sophisticated software creation possible for anyone with an idea.Meet the Agemo FoundersAymeric Zhuo, Co-Founder & CEOFrom Family Business to École PolytechniqueAymeric ZhuoScaling Gaming and Social Media GiantsAfter completing his Engineering and Applied Mathematics degrees at leading engineering schools including École Polytechnique in Paris, Aymeric joined Activision's media unit as a founding member working on all things data and analytics. There, he helped scale the business from zero to $350 million in revenue over four years, playing a crucial role in launching Call of Duty Mobile. At TikTok, he served as Senior Product Manager, where his work on creator monetization and algorithm optimization impacted hundreds of millions of users.Osman Ramadan, Co-Founder & CTOEarly Breakthroughs in SudanOsman RamadanLeading AI at MicrosoftAt Microsoft, Osman's four-year tenure transformed the company's machine learning initiatives. He led the federated learning initiative and spearheaded the Machine Learning and NLP Guild within Microsoft Mobile Experience, coordinating teams across London, Redmond, and Hyderabad. His work resulted in several widely-cited papers and patents in privacy-preserving machine learning and dialogue systems.A Lab Experiment Gone RightWhen Paths Align: The Lab StoryAymeric and Osman's paths crossed at an applied AI Lab founded by early DeepMind employees. While developing an AI-powered Instagram Post Generator in December 2022, they discovered a broader opportunity: the potential to program language models to generate and orchestrate software architectures. Both were drawn by complex and ambitious problems and they could foresee how building a "text-to-app" system would open up a wide range of opportunities to empower non-developers to create software.The Vision: Software Creation for EveryoneTheir shared vision for making software creation available for the many led them to turn down positions at OpenAI and found Agemo. Their complementary backgrounds - Osman's expertise in research on language models and privacy-preserving AI, combined with Aymeric's track record in scaling products to hundreds of millions of users - and commitment to building a generational company uniquely position them to tackle the challenge of enabling billions of people to create software.Dunia: Engineering Intelligence for SoftwareFrom a demo in the early days to an AlphaGo-like system for software reasoning, Dunia is the accumulation of months of research and development. Unlike traditional AI approaches that focus solely on code generation, Dunia reasons about software holistically - from system design to deployment - mirroring how experienced engineers approach and solve complex problems.About AgemoBacked by

Introducing Agemo
Aymeric Zhuo2024-11-06
Software is the universal language of innovation, powering everything from global enterprises to small businesses. Creating software, however, remains the domain of a select few — those with years of technical training and engineering expertise. Despite the advent of AI assistants like ChatGPT and GitHub Copilot, turning an idea into working software still demands deep technical knowledge.Agemo exists to change this paradigm. We envision a world where anyone can create sophisticated software tools simply by describing what they want to achieve. The future of software creation lies not in writing code, but in expressing ideas and problem solving.This idea would have seemed impossible just a few years ago. Since then, large language models (LLMs) have revolutionised the practice of writing code, finding a killer use-case in programming assistants and autocomplete. However, the fact that software creation remains stubbornly inaccessible reveals a deeper truth: Building software involves understanding requirements, designing systems, ensuring reliability, and managing deployment — aspects that demand human-level reasoning capabilities beyond the reach of traditional AI systemsThis insight drives our mission at Agemo.We're developing AI systems that can reason about software at a fundamental level — systems that understand not just how to write code, but how to transform ideas into reliable, production-ready solutions.These systems will be the foundational engine of our vision to bring software creation to everyone.The AI Status Quo for IntelligenceWith increasing scale, transformer-based LLMs have demonstrated remarkable abilities at a wide range of complex tasks, from answering medical queries to performing olympiad-level mathematical problem-solving. Within the software domain, these models impress with their ability to produce semantically and syntactically functional code, solve isolated programming challenges and provide coherent explanations for their solutions.Yet, something crucial is missing: the ability to reason about software as a complete system.While it is tempting to keep extrapolating and assume that AI-powered software creation will be solved by simply scaling up transformers to ever-larger datasets and parameter counts, their failure modes (remember This limitation becomes critical in software development. Consider how an experienced software developer approaches a new problem. They don't immediately start writing code. Instead, they think through the requirements, consider different architectural approaches, plan how to test and validate the solution, and design for maintainability and scalability. They reason about the software as a whole, understanding how different pieces will work together and anticipating potential issues before they arise.To emulate this kind of systematic reasoning, we must look beyond scale for a solution. The path forward lies in developing hybrid approaches that combine the pattern recognition strengths of neural networks with the rigorous reasoning capabilities of symbolic systems. This isn't just about making current approaches incrementally better. It requires rethinking how AI systems approach software development: Reasoning beyond Code GenerationDriven by our conviction that true software reasoning requires more than just pattern matching, we have developed We call this Reinforcement Learning from Machine Feedback.This methodology enables us to bridge the gap between human intent and working software in ways that pure neural systems cannot.Today, Dunia can take high-level descriptions of user requirements and transform them into fully functional backend systems. It handles everything from initial design through implementation, testing, and deployment — all while maintaining a clear chain of reasoning that can be inspected and verified. From automating repetitive workflows to building single-use APIs, While the scope of software it can generate today focuses on single-purpose backend systems, this represents just the beginning of our journey. Our research will continue to push the boundaries of what's possible in automated software development.The implications of this technology extend far beyond just making developers more productive. By abstracting away the technical complexity of software creation, this opens up new possibilities for how we think about and create software.Product manifestations of such a system will pave the way for a new class of software workers with a shift from writing code to expressing intent - from telling computers how to do something to telling them what we want to achieve. From “Software-as-a-Service” to “Service-as-a-Software”.Building AgemoBuilding a generational company starts with a special group of individuals. Our engineers, researchers, and product experts bring together expertise from leading institutions such the University of Cambridge, École Polytechnique, Imperial College, and The Alan Turing Institute, complemented by industry experience from Microsoft, Palantir, Meta, PolyAI, TikTok, and Goldman Sachs.But credentials are just one side of the equation. We index on grit over talent, we care about excellence of the craft, and we are relentless in our focus. We’re driven by a shared conviction that building truly intelligent systems requires more than following the status quo. Our approach to innovation is guided by three core principles:Systems over models.Owning the whole value chain.Data, network effects and velocity remain the moat.We are fortunate to be backed by This is just the beginning of our journey and the future cannot wait. If our mission resonates with you, we invite you to

It Has Never Been This Easy to Create Software
Hassan Mir2024-10-25
The way we build software is changing, and AI is leading the charge. Traditional development, once reserved for those with specialized coding knowledge, is being replaced by a new model where anyone can bring ideas to life. Platforms like Memeify: A Use Case for CodeWordsTo illustrate how CodeWords can be a game-changer, let’s walk through With CodeWords, I started by chatting with the architect—an AI-powered chatbot that helps you plan and design your software. After a quick conversation outlining my idea, it wrote my Function Spec. Once I confirmed it matched what I wanted, I clicked the Build button. The AI builder took over, and within minutes, the first iteration of the backend function was complete and deployed.The image illustrates the flow of building Memeify on CodeWords. The user starts by inputting a single sentence, then the AI Architect suggests a specification. After reviewing it, the user clicks "Build." The AI Builder takes the spec and, within a few minutes, delivers a fully deployed function ready to run. On the Run page, you can test the function. If you're not satisfied or need changes, you can simply return to the Architect and chat through your updates.Currently, CodeWords excels at creating stateless backend systems, making it perfect for projects like Memeify. Although the platform includes a lightweight user interface, I opted to implement my own frontend because of my familiarity with frontend development. I used a simple Next.js and React setup, quickly creating the buttons and image upload functionality.The workflow designed and implemented by the AI Builder.At first, I wasn't completely happy with the text layout on the meme, so I iterated on the design. I copied my spec into the CodeWords architect and asked it to split the text into two parts. I wanted text positioned at both the top and bottom of the image, using the classic Impact font for memes. The architect made these adjustments seamlessly, letting me refine the design until it was exactly what I wanted.Following the codewords-client The Memeify Website.Speed, Efficiency, and AccessibilityThe ease and speed with which CodeWords enables software creation is a game-changer. It’s not just about making development faster—it’s about enable and encourage more people to bring their ideas to life. With AI handling the heavy lifting, you can iterate and experiment rapidly, making development more agile and responsive.And it doesn’t stop there. Agemo AI’s CodeWords Consultants service takes it a step further, offering real-person assistance. Just share your project idea, and a consultant will build the backend for you using CodeWords. It’s a new level of convenience and support that democratizes software development even further.Cost-Effective DevelopmentBuilding this function on CodeWords cost roughly 50 cents, covering all the AI model usage. Each execution costs mere millicents—about 1,000 executions for just a dollar. With a little monetization, such as ads, projects like Memeify could easily turn profitable. But for me, this was just an opportunity to showcase what can be done with CodeWords.What Will You Build?AI is redefining software development. With CodeWords, we’re making it faster, easier, and more accessible than ever before. The question now is, what will you build? The future is in your hands, and it’s only one prompt away.